This post has been on my mind since 2023. I had just started at a company that used AI as the user experience differentiator. We were building ambitious features, but I kept noticing that when teams wanted to add AI, their first instinct was almost always: “Let’s add a chat copilot!”
It makes sense on the surface. The chat feels flexible. You can ask anything; it adds AI magic to an existing product. But here’s the thing: the obvious first step isn’t always the best. Chatbots, while neat, come with a hefty dose of UX headaches. It’s time for us, as designers, to start thinking beyond the chat.
The Chatbot Conundrum: Shiny Toy or User Frustration?
Chatbots give you flexibility: ask away, get info, and do stuff without clicking a million buttons. Sounds great. But, it’s not all sunshine and rainbows:
-
Discoverability: You drop a chatbot onto a screen. How does anyone know its superpowers? Unlike a nice, clear button that says “Export Data,” a chat box is a blank slate. Users end up playing a guessing game, get frustrated, and -maybe- ignore it.
-
Hallucinations: AI can be confidently wrong, especially when trying to understand the specific world of your app. It may invent an answer that sounds right but is off. That’s not just annoying; it can break trust or cause real problems.
-
Speed: LLM isn’t magic pixie dust speed. The AI needs time to think, call out to other tools or APIs, and then spit out an answer. That lag feels clunky compared to the instant feedback we expect from most apps.
-
Costs: Building the chat might feel cheaper upfront, but running it involves costs. Every question uses compute power (LLM tokens) and maybe API calls. Users often have no idea they might be incurring a bill due to lengthy contexts or expensive API calls.
-
Context: Does the AI know you’re asking about the specific report you’re viewing or your entire account history? Getting the context right and ensuring the user understands the scope given to the AI is surprisingly complex.
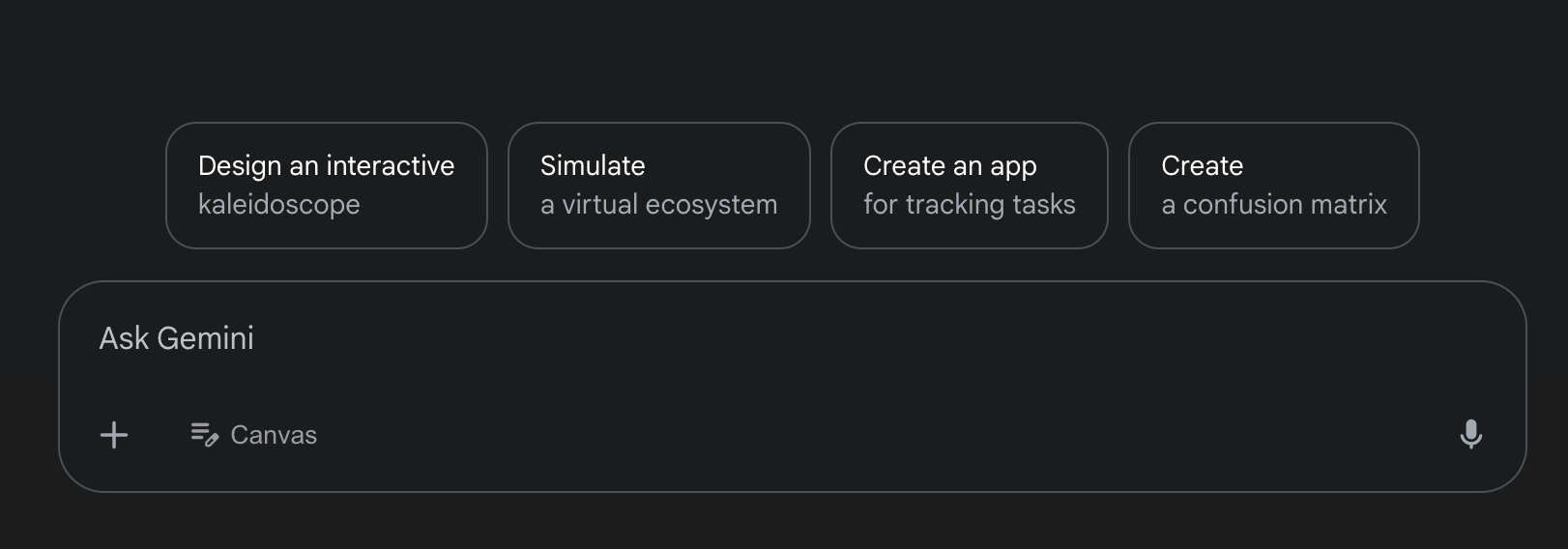
Providing prompt examples is a common practice to demonstrate the capabilities of a chatbot.
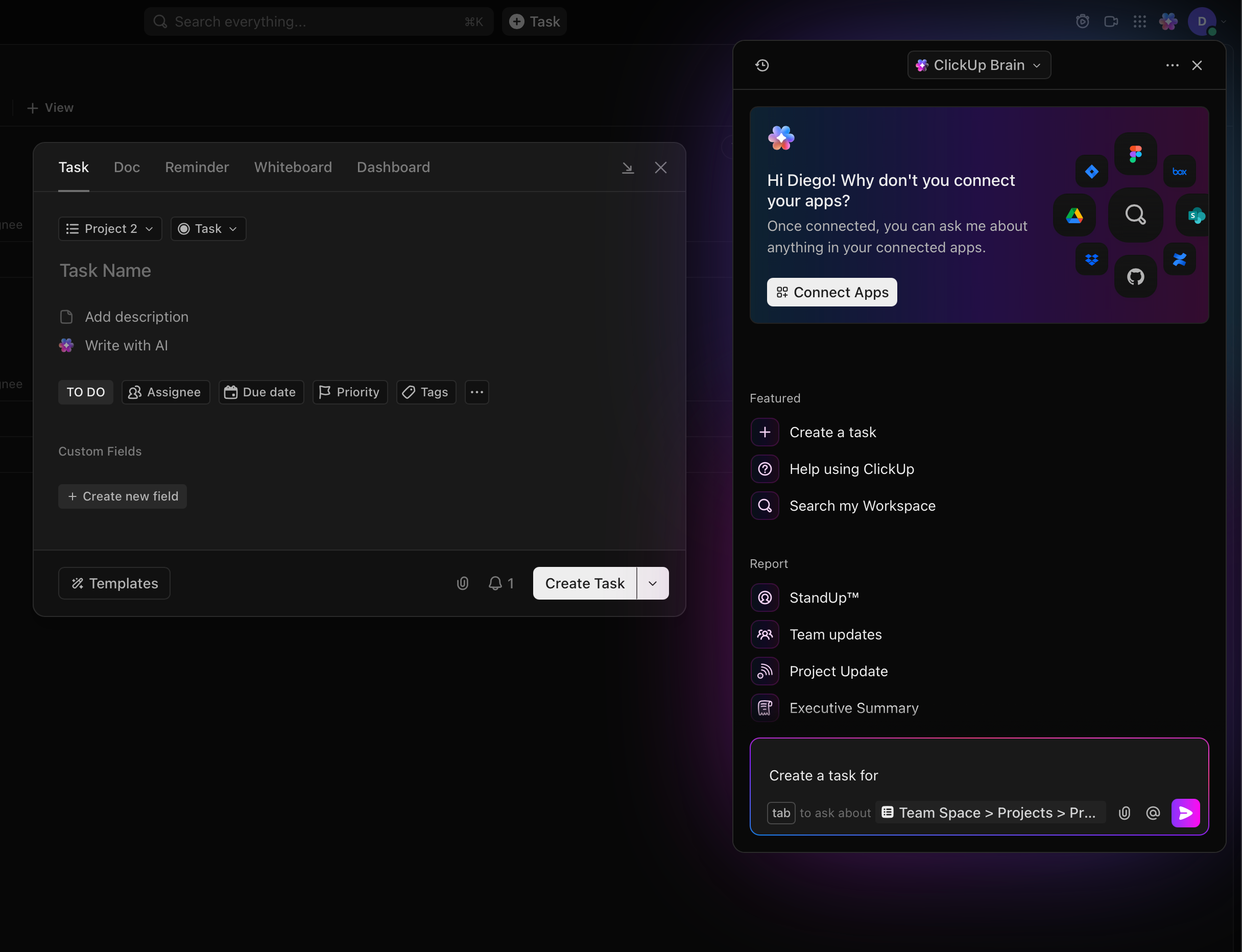
Click-Up’s user interface uses multiple UI patterns. The chatbot’s task functionality highlights the primary challenges chatbots face: when I provide the task information to the chatbot, will it create the task in the right list? The non-AI version is faster and more reliable.
Gemini Flash 2’s response appears accurate and confident, but is incorrect (newer versions of Claude, ChatGPT, and Gemini provided the correct answer). AI hallucinations can occur regardless of the user interface, but prompts written in a certain way can increase their likelihood.
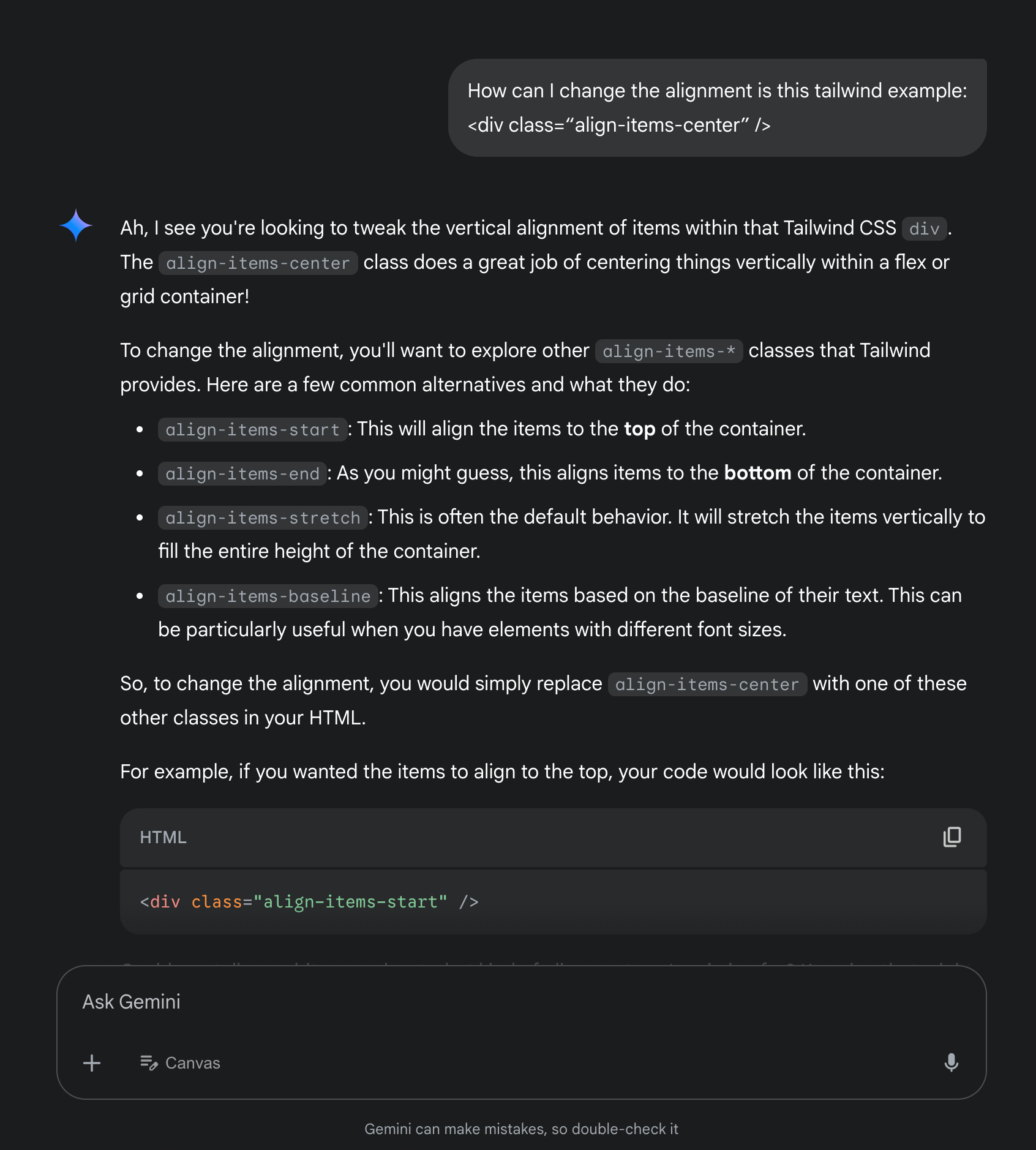
What’s the Catch with Not Using Chat?
What about building AI features directly into the flow? Well, that path has its bumps:
-
Flexibility: When AI is built-in for specific tasks, such as summarizing selected text, it’s usually more predictable. But you lose that “ask anything” vibe. Users can’t easily explore or use the AI in ways you didn’t explicitly design for. It’s a trade-off.
-
Speed and Expectations: You can often optimize built-in AI features more effectively than a general chatbot. However, complex AI tasks still take time. We need to get good at showing users something is happening (spinners, progress bars, gradual reveals) so they don’t just stare at a frozen screen.
-
More Design Sweat (Investment): Adding a chat widget is one thing; designing an integrated AI feature requires user research, prototyping, testing, and iteration.
-
Keeping the AI on Rails (Output Control): We want AI to be helpful and predictable. We can tell LLMs to give us answers in a specific format (like JSON), but tightening the reins too much can kill the user’s creative spark. It’s a balancing act.
-
Selling It: A chatbot screams, “Look, we do AI!” It’s an easy marketing win. More subtle, integrated features might be better UX, but they don’t shout as loudly. Explaining the differentiator takes more finesse.
Emerging Patterns: Beyond the Basic Bot
The good news is that we’re already seeing clever ways AI is showing up without a chat window hogging the screen or by blending chat with other interactions:
-
Inline Assistance: Think Notion AI, Grammarly, or the features Apple showed for macOS Sequoia. You select the text, and boom - options pop up to summarize, rephrase, and correct grammar right where you are. The AI helps you accomplish tasks without context switching.
-
Guided Creation: Apple’s Image Playground is an attempt at this. Instead of making you a prompt wizard, it provides menus: choose a style, a subject, and a setting. It guides you in creating something, lowering the barrier for everyone (yeah, Image Playground is not the best example of execution and usefulness).
-
Supercomplete: Tools like GitHub Copilot or Cursor don’t just finish your line of code; they look at the context and suggest whole blocks of code. It feels like they’re anticipating your next move.
-
Chat + Canvas Hybrids: We also see interfaces that blend conversational interaction with direct manipulation. Think about environments like Google’s Gemini Canvas - you can chat with the AI to generate content, such as text or code. Still, you can directly edit, refine, and rearrange that content in the adjacent workspace. It combines the flexibility of chat for initial generation with the control of a traditional editor for iteration.
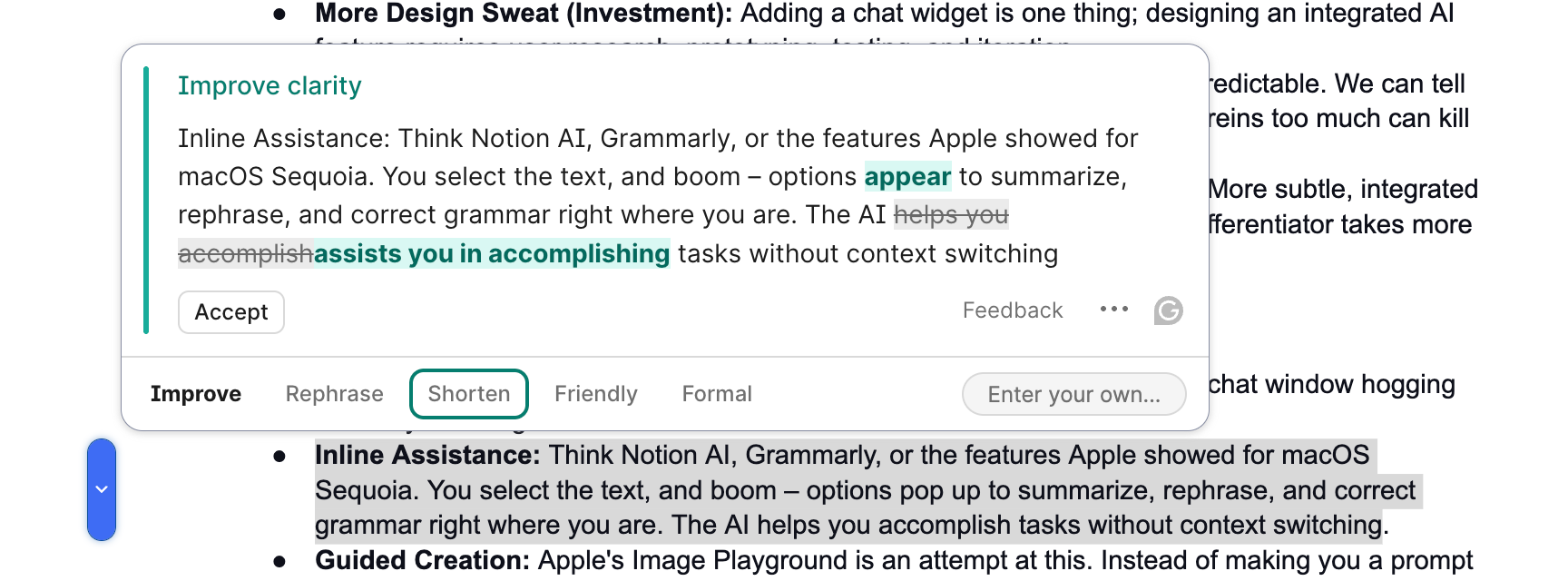
The Grammarly plugin for Google Docs follows a similar pattern to many other AI writing assistant tools. I like how they improved the AI suggestions and corrections over the releases. Although not very discoverable, you can click on the highlighted corrections to accept or reject the changes.
The Apple Image Playground allows users to generate images without prompts. While the concept and blob animation are pretty, the execution has been disappointing. The generated images are often subpar, revealing limitations of the underlying DALL-E model. The straightforward interface exposes these weaknesses more clearly than a chatbot format would.
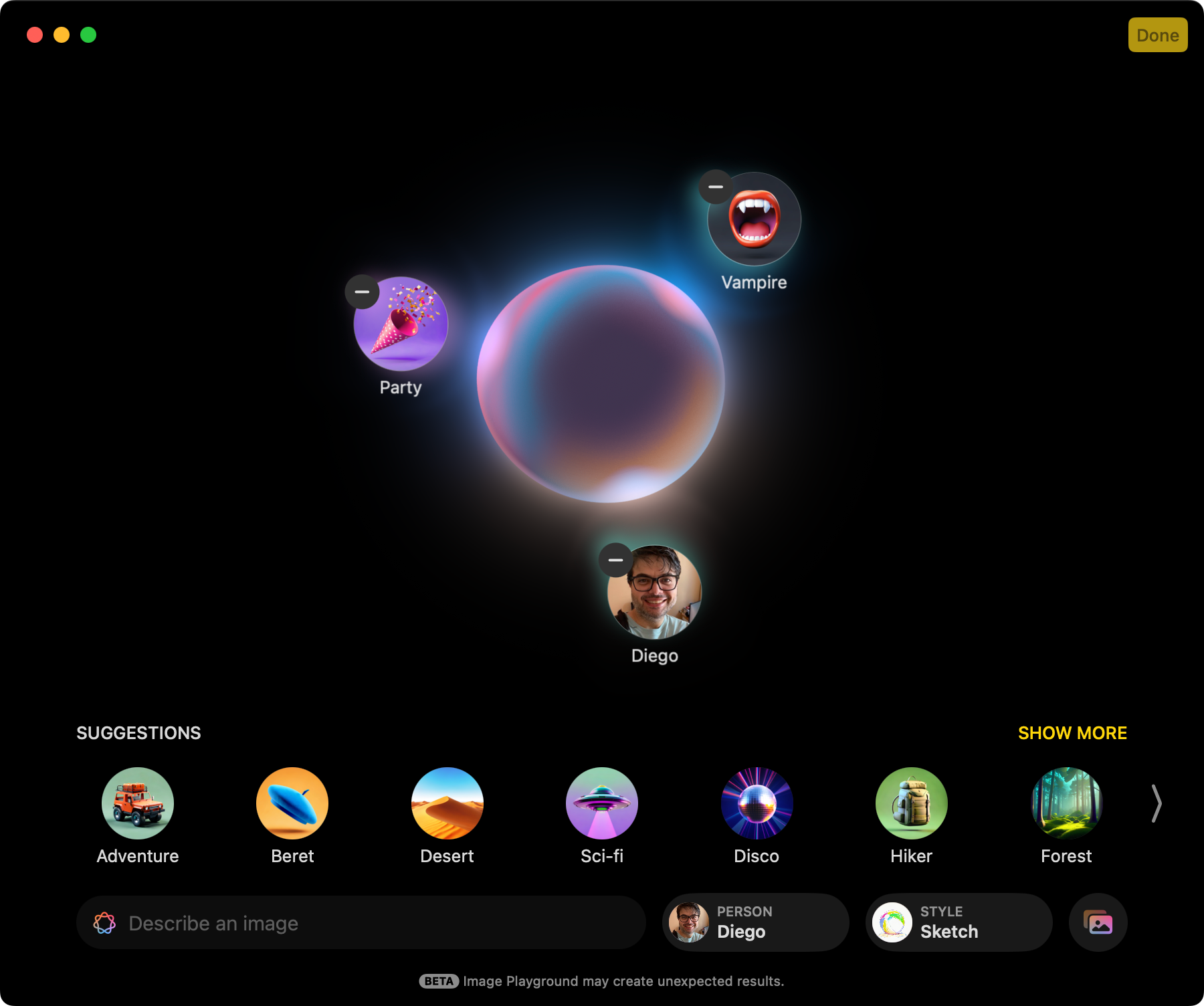
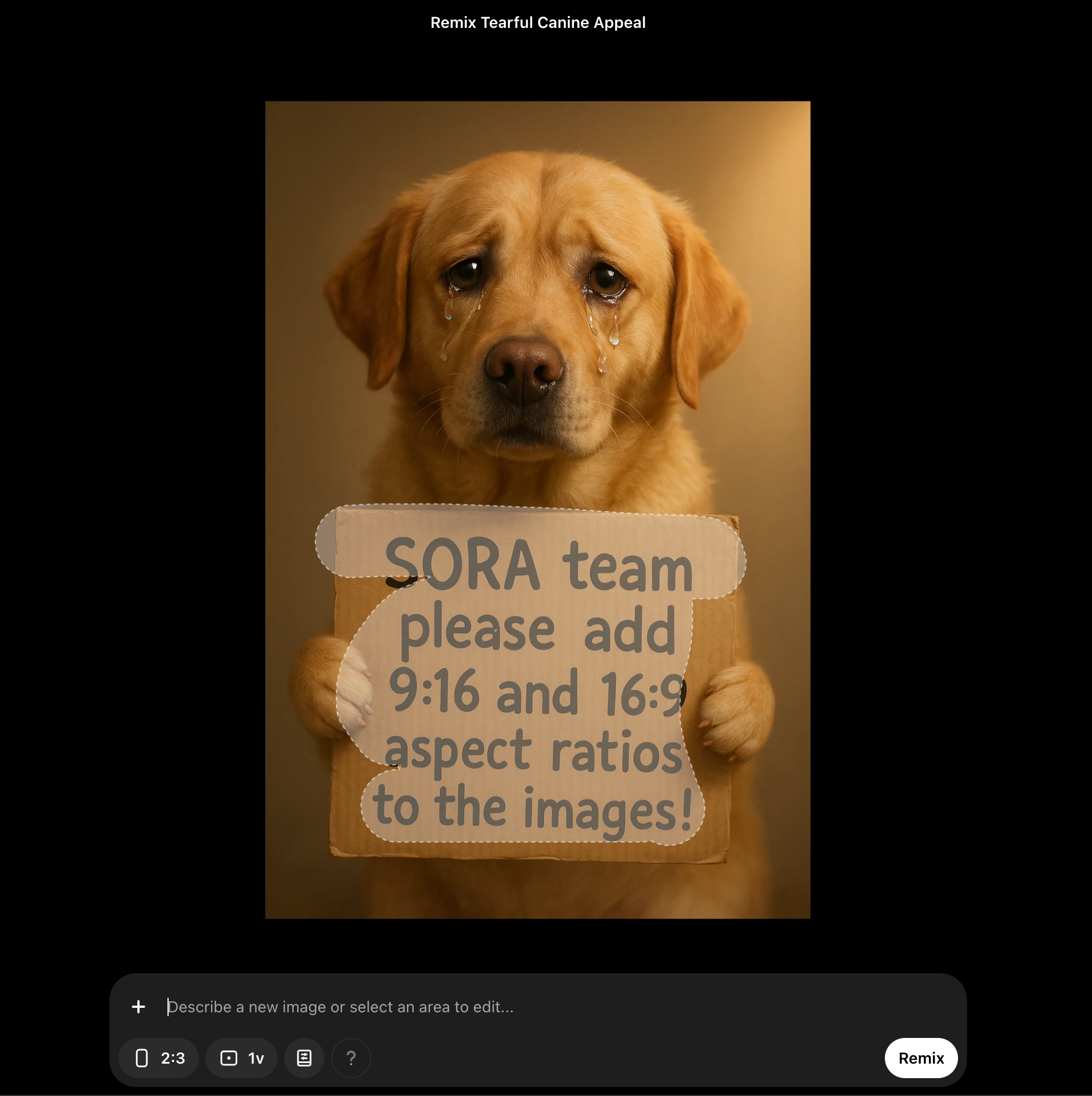
The remix functionality of OpenAI Sora has a hybrid interface where you can highlight regions of the image and write prompts. Other AI image editors have used this approach, which is also common in AI IDEs. As applications adopt AI, we’ll see hybrid UIs more often.
Is the OS the New Chatbot?
Here’s another twist: AI is getting baked into the operating system. Think Microsoft Copilot or Apple Intelligence. These big-brain AIs want to understand what you’re doing across all your apps.
With plugin systems (like MCP — Model Context Protocol), the idea is that your app might not need its chatbot. Perhaps it just needs to tell the OS assistant, “Hey, here’s my data, and here’s what I can do.” Then, users interact with one central AI. This raises the question: Do we build our bot or plug the app tools into the OS chatbot?
As of April 2025, this OS-level functionality is still half-baked but is moving quickly. Don’t be shocked if Apple, Microsoft, or Google make big announcements soon about deeper AI integration in their systems.
What features can we expect in future OS versions?
-
Tighter automation framework integration: This is somewhat present (Siri, Cortana, and “Ok Google” can launch shortcuts and apps), but not as closely integrated with an AI assistant as MCP tools.
-
Native chat UIs, possibly well integrated with the web browser. Microsoft took the first step with Edge Copilot, but costs and privacy concerns have hindered its progress. Local LLMs may address this, though they often require robust hardware.
-
Screen recognition-based automation. Microsoft, OpenAI, and Anthropic have trialed this kind of agent.
-
SDKs to expose and index data for AI agents: while these exist, I anticipate software vendors will standardize and simplify their platform-specific frameworks. Various small RAG tools exist; this functionality aligns well within the OS.
-
SDKs to execute actions in an isolated environment. We can do that today with containers (eg, Docker), but with the rise of agentic UIs, sandboxing, undo, and preview of actions, it’s a common expected feature that the platform can solve.
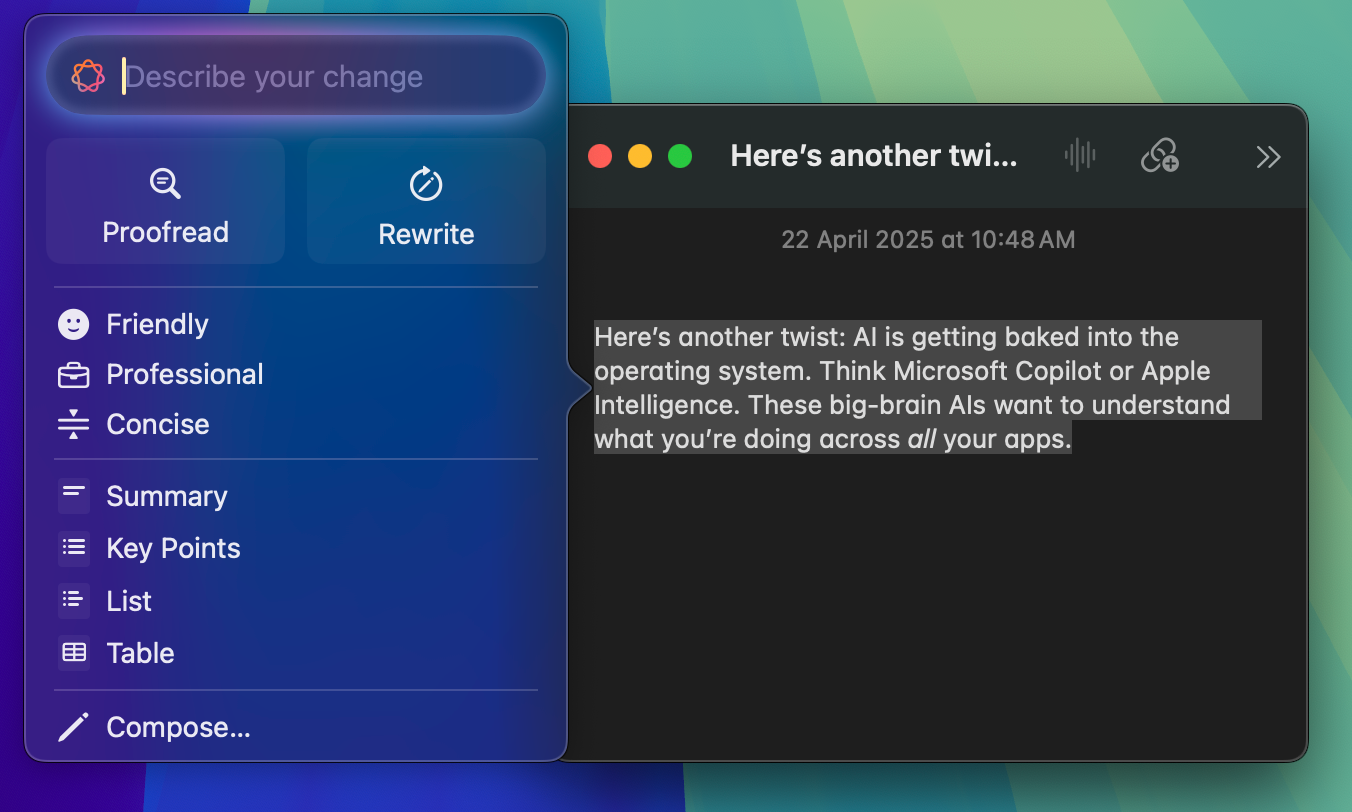
The recent release of macOS Sequoia included AI writing tools available for every native app. While it’s possible to launch ChatGPT to compose text using the writing tools, there is still much room for improvement and possible features.
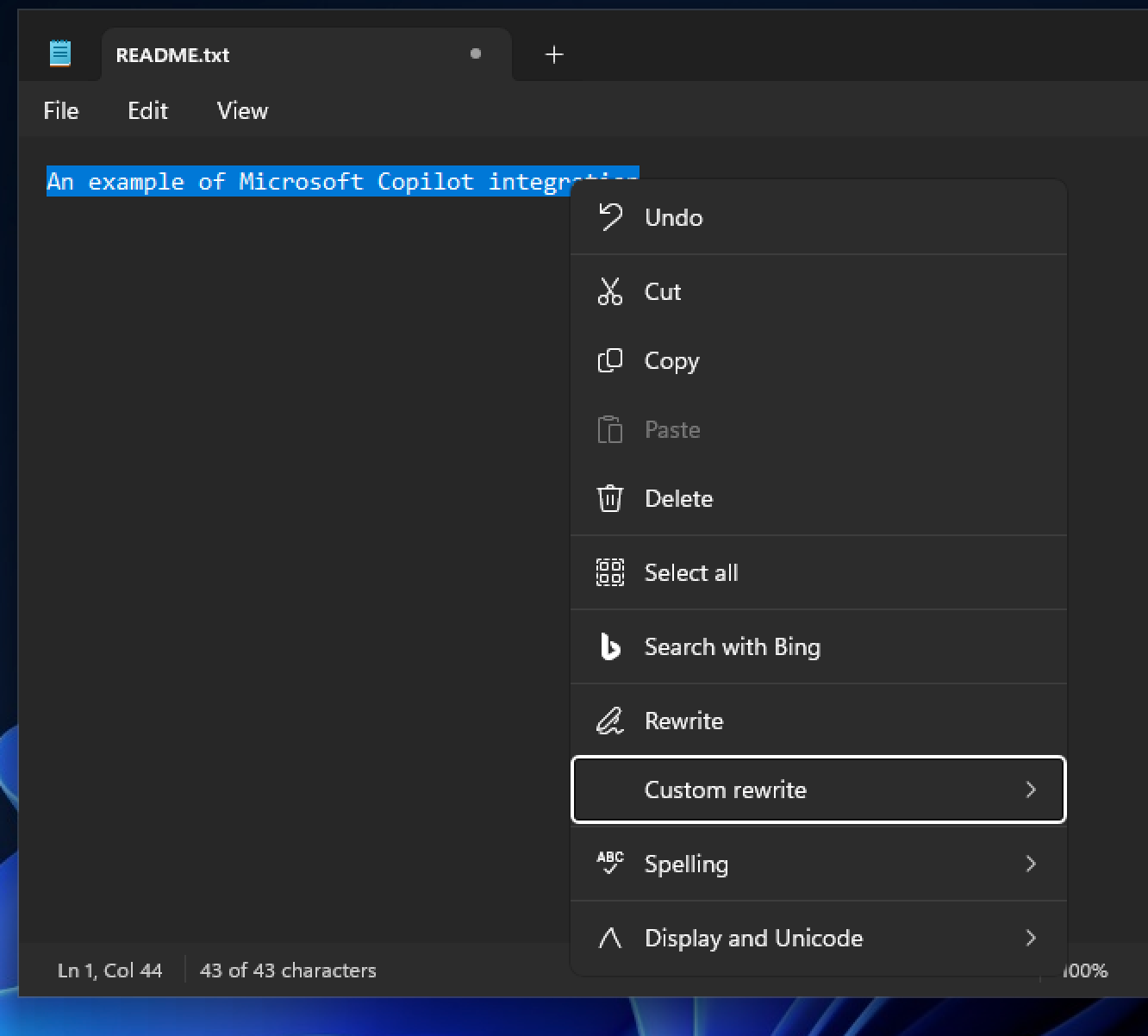
Microsoft also included AI rewrite features in its latest version of Windows.
Designing the Future: Our Role
Often, the first wave of a new technology is just about making the tech work. The next wave is about making it work for people. That’s where we come in. As UX folks, our job is critical:
-
Beyond chat: Question the chatbot default. Is it the best tool for this specific user problem? What else could we do?
-
Core UX: All the stuff we know about UX (user research, journey maps, testing, etc.) is more critical now. AI needs to be intuitive and discoverable, not just clever.
-
Generative Potential: How can AI create new experiences? Dynamic interfaces that adapt? Personalized workflows? Content generation that helps? Let’s get creative.
-
Microinteractions for AI: How do we show how AI is thinking? How do we handle uncertainty? How do we make waiting less painful? The little details matter hugely for trust and usability.
Chatbots aren’t going away entirely. They’ll have their uses. But the truly great AI experiences will be woven seamlessly into the fabric of our tools, helping us out in natural and innovative ways.